The Future of Decentralized AI: Opportunities and Challenges
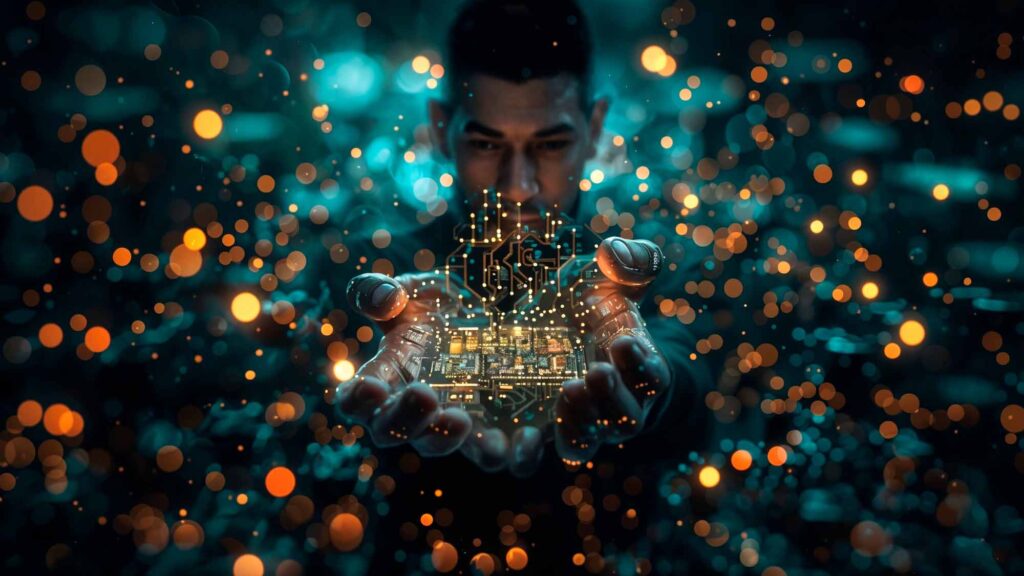
As artificial intelligence (AI) continues to permeate various aspects of our lives, the concept of decentralized AI is gaining traction. By integrating AI with blockchain technology, decentralized AI aims to address concerns related to data privacy, control, and equitable access. This blog post explores the promising opportunities and inherent challenges associated with decentralized AI.
Opportunities in Decentralized AI
- Enhanced Data Privacy and Security
Decentralized AI frameworks, such as federated learning, allow for model training across multiple devices without the need to centralize data. This approach ensures that sensitive information remains on local devices, reducing the risk of data breaches and unauthorized access. By keeping data decentralized, users maintain greater control over their personal information.
- Democratization of AI Access
Traditional AI development is often dominated by large corporations with substantial resources. Decentralized AI seeks to level the playing field by enabling individuals and smaller organizations to participate in AI development and deployment. This democratization fosters innovation and allows for a more diverse range of AI applications tailored to various needs.
- Improved Transparency and Trust
Blockchain technology, a cornerstone of decentralized AI, provides an immutable ledger of transactions and processes. This transparency enhances trust in AI systems, as stakeholders can verify the integrity of data and algorithms. Such openness is crucial in applications where accountability and auditability are paramount.
- Resilience and Fault Tolerance
Decentralized AI systems distribute workloads across multiple nodes, reducing reliance on a single point of failure. This architecture enhances system resilience, ensuring continuous operation even if individual nodes encounter issues. Such fault tolerance is vital for critical applications requiring high availability.
Challenges in Decentralized AI
- Scalability and Performance
Coordinating AI processes across a decentralized network can introduce latency and computational overhead. Ensuring that decentralized AI systems scale effectively while maintaining performance is a significant technical hurdle. Optimizing communication protocols and resource allocation is essential to address these concerns.
- Data Heterogeneity
In decentralized settings, data sources can vary widely in format, quality, and distribution. This heterogeneity complicates model training and may impact the accuracy and generalizability of AI models. Developing techniques to harmonize diverse data inputs is critical for effective decentralized AI.
- Governance and Standardization
Without centralized oversight, establishing governance frameworks for decentralized AI is challenging. Determining responsibility, enforcing ethical standards, and resolving disputes require collaborative approaches and consensus mechanisms. Standardizing protocols and practices is necessary to ensure interoperability and trust among participants.
- Regulatory Compliance
Navigating the complex landscape of data protection laws and regulations poses challenges for decentralized AI. Ensuring compliance across jurisdictions requires careful consideration of legal frameworks and the implementation of privacy-preserving techniques. Balancing innovation with regulatory adherence is a delicate endeavor.
Conclusion
Decentralized AI represents a paradigm shift in how AI systems are developed, deployed, and governed. By leveraging the strengths of blockchain technology, it offers promising solutions to longstanding issues in AI, such as data privacy, access inequality, and transparency. However, realizing the full potential of decentralized AI necessitates addressing technical, ethical, and regulatory challenges through collaborative efforts and continuous innovation.